Loading data into Redshift with DBT
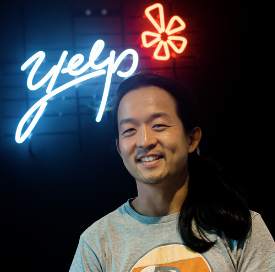
-
Christopher Arnold, Software Engineer
- Nov 6, 2024
At Yelp, we embrace innovation and thrive on exploring new possibilities. With our consumers’ ever growing appetite for data, we recently revisited how we could load data into Redshift more efficiently. In this blog post, we explore how DBT can be used seamlessly with Redshift Spectrum to read data from Data Lake into Redshift to significantly reduce runtime, resolve data quality issues, and improve developer productivity. Starting Point Our method of loading batch data into Redshift had been effective for years, but we continually sought improvements. We primarily used Spark jobs to read S3 data and publish it to our...
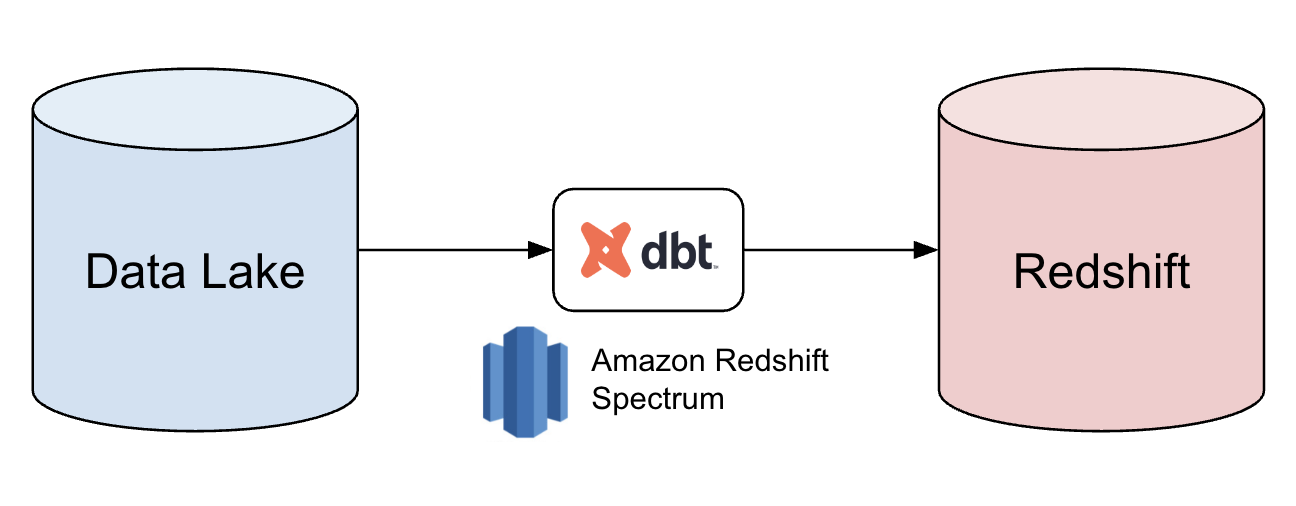