How we improved our Android navigation performance by ~30%
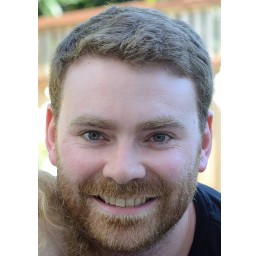
-
Paul Martin, Core Android Tech Lead
- Oct 8, 2024
In 2019, Yelp’s Core Android team led an effort to boost navigation performance in Yelp’s Consumer app. We switched from building screens with multiple separate activities to using fragments inside a single activity. In this blog post, we’ll cover our solution, how we approached the migration and share learnings from along the way as well as performance wins. Where we started circa 2018 Navigating between screens in an Android app is often when the app and device are under the most strain. The new screen and its dependencies are quickly created, which can lead to slow or frozen frames. Prior...
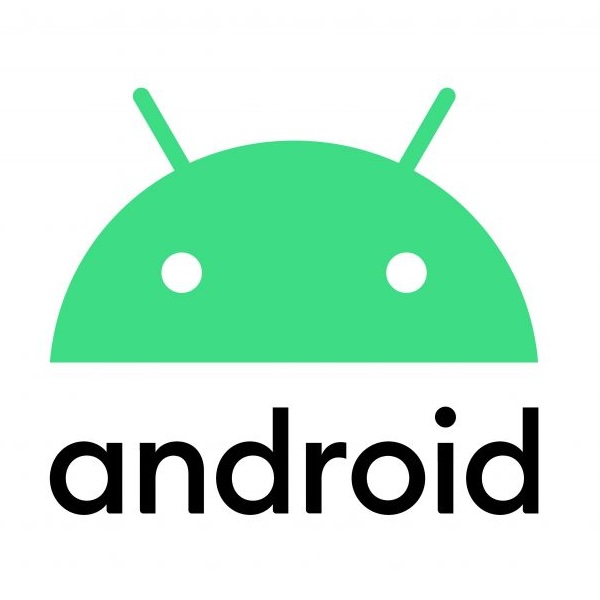